Integrating Generative AI with Cloud Platforms Technical Considerations and Implementations
Generative AI Consulting has risen to set the spotlight as firms seek to leverage contemporary technology in operation. Over time, cloud platforms have become the perfect home for generative AI solutions since they support both scalability and modularity while reducing costs. However, integrating generative AI with the use of cloud services is not without mostly technical challenges.
There are several issues that businesses/organizations have to sort out before deployment in terms of data security and performance optimization. We also discuss here the main technical features and approaches to covering them that allow for effective integration with cloud services for generative AI.
Acknowledging the Benefits of Cloud for Generative AI
The benefits that cloud platforms provide to firms are numerous in the case of generative AI services, hence why businesses turn to cloud platforms:
- The clouds offer flexibility, for an organization can quickly add or shrink depending on the need, something that is important for AI, which is sometimes unpredictable depending on the load.
- Cloud platforms are flexible in deployment and provide an opportunity to deploy AI models in different settings.
- The cost-efficiency of cloud infrastructure plays an important role in the cost-effective management of companies’ AI activities, and they do not need to spend a lot on hardware.
- Cloud providers currently provide solutions for generative AI to support the optimization of processes and reduce developers’ workload.
In general, the cloud platforms offer the required infrastructure for an effective Gen AI Consulting framework.
Purpose: To Identify the Key Technical Factors That Need to be Taken into Consideration When Integrating Generative AI Solutions in Organizations
While implementing generative AI with cloud platforms, there are key technical decisions that are significant for successful integration. The first challenge is safety, as the AI model can, in most cases, need large records that might be sensitive. Any civil platform must be compliant with all data protection regulations of the cloud platform.
Another factor is latency since AI services that need to operate in real-time must be performed with minimal delays for the purpose of returning accurate results as soon as possible. Cloud providers provide different regions and edge computing solutions to help reduce latency problems.
Selecting the Most Suitable Cloud System for the Deployment of AI
The choice of a correct cloud platform is vital when considering the process of running generative AI. Thus, every cloud provider has its characteristics as well as the services that are beneficial for AI systems. For instance, Aws, Google Cloud, and Microsoft Azure, among others, have additional services with AI and machine learning properties that will help to improve the results of the models.
Combining the Use of Pre-Trained Models and Designing Solutions From Scratch
This is one of the most critical and major choices to be made when deciding on using generative AI, whether to use ready-made models or build models from scratch. Learning models that are available in the cloud are superior to the pre-built models, as the cloud providers have optimized them for certain schemes.
Improving AI Workflows via Using Cloud Platforms
Today, cloud platforms provide numerous tools that facilitate the work of AI from the initial stage of development to the deployment of AI systems. For instance, AWS and Google Cloud present precepts such as TensorFlow and PyTorch, which make it easy to train models.
Supervising and Controlling AI Model Efficiency
If generative AI models are in the cloud environment of the organization, then it is essential to watch and control their execution closely. Cloud providers provide a wide array of monitoring solutions that assess different aspects of performance, including the time, accuracy, and resources needed.
These tools assist businesses in identifying areas of concern as they occur, thus enabling them to respond quickly. Also, cloud platforms offer scalable solutions to ensure that resources are automatically set depending on the predictive modeling results and needs. This means that the AI services can continue executing their workloads even in the face of variations in workloads.
Discussing Difficulties in the Application of Generative AI
However, there are also some issues when integrating generative AI with cloud platforms, as follows. Data privacy is the first issue because of the voluminous data that are usually processed and analyzed, and these data may be in the form of personal data.
There is a major need to adapt to good encryption measures and follow the rules provided in the data protection legislation. In the same regard, dealing with the complexity of models is also difficult, as the more complicated models could demand much computational power and may also be hard to maintain.
Conclusion
Integrating generative AI with cloud platforms offers businesses enormous opportunities. However, it is a complex process that requires strategic planning and efficient technology control. Generative AI consulting’s main expertise is helping companies manage the implementation issue, which is critical for most businesses. Key areas include the cloud, data protection, and model refinement.
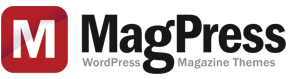